Automation and productivity are at the forefront of priorities when it comes to businesses that provide data reconciliation services. Data integrity plays a significant role as a foundation to better optimize the reconciliation lifecycle. Business Efficiency and Data Management as a whole have become important for the reconciliation process as the data is constantly being extracted and further processed from a number of different sources.
There are many steps to ensure that the quality of Business Efficiency and Data Management is optimal to use for business insights. Let’s discuss some key features and approaches to data integrity and how one can provide their business with quality source data.
Table of Contents
Improvements in Business Efficiency and Data Management
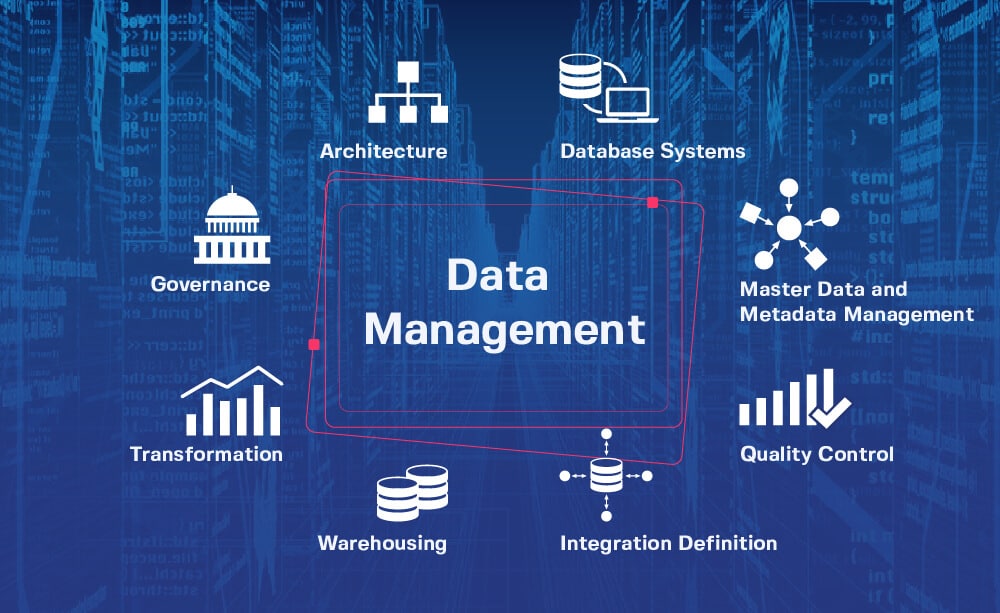
Ensuring Data Quality for Better Data Insights
A well-informed data quality strategy includes a number of processes and outcomes. These are facilitated by maintaining a dashboard for data quality that help owners of the data and IT support in gauging the measurement for data quality. This dashboard also facilitates taking forward processes of simple data cleansing and validation rules. The dashboard ensures that there is a central space where all inconsistencies in the data are swiftly identified between systems.
The data reconciliation processes require formalization, which in turn helps in ensuring that the processes are performed as scheduled or on an ad-hoc basis. There needs to be increased visibility into the reconciliation process and the results that they garner. This helps in enhancing the overall confidence of the business user, and decisions can be consecutively made with ample system data backing them up.
Data quality activities can be controlled by defining a proper set of data quality metrics. Alongside this, Business Efficiency and Data Management have required the right validation rules, and further monitoring of data by using various data profiling tools and processes. Enterprise governance needs can be addressed by collaborating with other data stewards in the business.
Improve Data Management in Reconciliation Process
Some key challenges to data reconciliation include ensuring an environment where Business Efficiency and Data Management quality is a priority. This means that data quality policies and standards across enterprises need to be adhered to.
Top Data Analytics Companies are instances in the reconciliation process where incoherent or incomplete data is rejected by the environment as an error. These errors occur due to non-standardized data formats, changes in data sources, or frequent changes made to the reference data.
It is also important to have mentoring staff with knowledge of best practices when it comes to data quality and data handling. Operational lead time must be reduced so the data can travel from acquisition to insight in the shortest time possible. This ensures that there is a smaller marginal cost.
Let’s look at some steps to follow for a smoother Business Efficiency and Data Management reconciliation experience:
- Data managers can filter their data and increase transparency by using automated extraction using scripts, ETL and APIs.
- A comprehensive and detailed audit of the business can help the efficiency of the data reconciliation process.
- Maintaining a historic data repository can help in making the reconciliation process faster. This can be achieved by opting for independent control in order to balance, reconcile, and track real-time information.
- Data can sometimes be lost in data silos or in data graveyards which can lead to hidden or inconsistent data. For example, data about clients that the Sales team has information on may not be shared with the customer service team which may lead to a loss of opportunity to create more distinguished and accurate customer profiles.
- Data downtime can cause various migration issues. This is caused by increasing the complexity and magnitude of data in the pipelines. Employing accountability by putting in service level agreements can help both the IT service provider and the customer in making sure there is less data downtime.
Fixes to Data Quality Issues
Data quality issues arise because of inadequate monitoring and management of Business Efficiency and Data Management in real-time. These include duplicate data, inconsistent formats, incomplete data, inaccurate data, and data imprecision. Here are some tips and best practices to address these issues for Business Efficiency and Data Management:
The right place to address any data quality issue is at the originating source system. A business process layer usually requires a higher level of intervention similar to that of an instance when the data is provided by a third party and there is no immediate control. Fixing data at the source is often the best way to make sure data goes through requisite data checks and gets cleansed and transformed at the entry stage itself.
Fixing the problem at the source can be difficult, so the process to fix data quality can be employed in the ETL process. This is what most businesses end up doing since it is a pragmatic approach. With the help of set algorithms and smart rules, clean data sets are derived that provide better business insights.
Managing metadata can go a long way in providing a business with clarity regarding business objectives. Common data definitions that are used across the enterprise across subject domains can be cataloged so as to provide a format and structure to the data quality initiative.
Data profiling allows for a deeper understanding of data where the frequency and distribution of data values are assessed in a Business Efficiency and Data Management set. This can be used to check data integrity and also helps determine whether the data conforms to business rules and defined data standards.
Quality Data for All Business Efficiency and Data Management
The business world has increasingly become more data-driven in the last few years. For this very reason, it is important to adopt the right data quality tools. Traditional methods of data reconciliation are getting both inefficient and non-sustainable in a fast-changing business environment. There is a need to put in place a data governance framework that will lay out the various data policies, standards, and data quality key performance indicators (KPIs).
The organization’s structure and roles need to be delineated so as to ascertain who the data owners are, the data custodians, and the data stewards. When implemented as discussed here, Business Efficiency and Data Management reconciliation will ultimately help improve the business user’s capabilities with data quality, product engineering services, data integrity, and accuracy.
Read more: Top Fintech Apps For Investment And Financial Operations